Hamid Omid is our AI agency partner. With over a decade of data science and software engineering experience, he helps companies identify meaningful big data and machine learning use cases and corresponding solutions to stay competitive. As more organizations look to integrate AI into their digital products and services, Hamid shares his expertise to help early adopters' projects succeed.
Plagiarism detection, biometric facial recognition, and movie recommendations are some of the ways in which we are already interfacing with artificial intelligence (AI) and machine learning (ML). As AI and ML gain popularity, companies and individuals are bursting with excitement to dive into projects of their own.
In 2017, firms around the world spent over $21.8 billion on mergers and acquisitions related to AI. While we are still at the beginning of the curve, McKinsey predicts that digital innovations will provide an estimated economic impact of about $370 billion per year worldwide by 2025. There is a significant opportunity for early adopters to capture an outsized share of that value, but not everyone can win. With a flurry of AI projects being funded, caution needs to be exercised when choosing which ones to pursue.
The stakes are high
Forbes recently reported that many executives worldwide are not yet seeing value from AI investments made in recent years. According to Harvard Business Review (HBR), 77% of executives say that business adoption of Big Data/AI initiatives is a major challenge, which is up by 65% from the previous year. These million and billion-dollar projects are failing to deliver on their promises, and we don’t want yours to be one of them.
Ask the expert
At integrityCo, our team has over 60 years of collective experience with machine learning, big data, data science, and software development—so when it comes to AI projects, we’ve seen almost everything. As the industry grows and more companies invest their dollars, we thought it would be helpful to share four common pitfalls and potential solutions to give your projects the best chance for success.
1. Team misalignment
All projects, especially innovative ones, need the right team and alignment among stakeholders. Without it, you are fighting an uphill battle. According to an HBR survey, 93% of respondents identified people and process issues as major obstacles to success. The challenges with culture change have been dramatically underestimated in leading companies. HBR found that 40.3% identify a lack of organizational alignment, and 24% cite cultural resistance as the primary factors stifling business adoption. Consequently, these are also negatively impacting the return of investment for AI projects.
Solution: Communication is key
Diverse perspectives are only valuable when team members have a chance to share them. Therefore, giving every person an opportunity to voice their opinion is critical to the project’s success. We recommend beginning your project with a workshop. By facilitating an engaging brainstorming session, people not only feel comfortable sharing their thoughts, but it also gets stakeholder buy-in, creates alignment, and saves time in the long run.
2. Quantity over quality
Data is critical to making informed decisions on AI projects. Often in the interest of saving time, many companies approach data gathering with the mindset that more is better. Unfortunately, this results in teams feeling overwhelmed and unsure how to parse through hundreds (sometimes thousands) of datasets to determine what is useful. In conducting data analysis for clients, we have come across terabytes that provided little insight into their problem and megabytes that were so rich with information we could build a sophisticated ML project. The key is to understand the problem your team is trying to have AI and ML solve, and collect quality data targeted at that solution.
Solution: Take small steps, and when in doubt, outsource
To determine relevant data, start with a small and easy exploratory analysis. Use what is readily available to determine if you can pull out any signals. A seasoned data scientist will be able to identify patterns, but if they can’t, try training a simple naive model as an alternative. You may need to start over with new parameters if there are still no clear signals emerging.
Developing an AI product is resource-intensive, and for many organizations, it’s challenging to allocate the in-house time and talent to work on every facet. Hiring a consultant with relevant domain and data science experience can be a great investment to ensure you collect quality data.
3. Irrelevant KPI + small impact
We’ve seen clients get swept up in fun and interesting projects only to find out the business value is centered around vanity metrics. For example, clients measure an increase in visitors when their focus should be increasing ARPR. Even if a project is tied to a relevant KPI, the effort required to make an impact renders some ventures more valuable than others. Passion projects are important, but before taking one on, first, determine relevant KPIs and the amount of impact it will generate for your business.
Solution: Know your business
To determine relevant KPIs, you need to know your business—this may sound trivial, but many companies can’t articulate the problem they are solving. Get your decision-makers together and ask:- What are we trying to solve?- For what purpose? - And what impact does it have on our business? Community?
As you are going through this exercise, think of ROI, not COI (coolness of investment!).
A tip for calculating ROI: ROI can be determined by looking at how much you stand to make or save if the project succeeds. The example below demonstrates how you might go about forecasting ROI for a potential affiliate marketplace project.
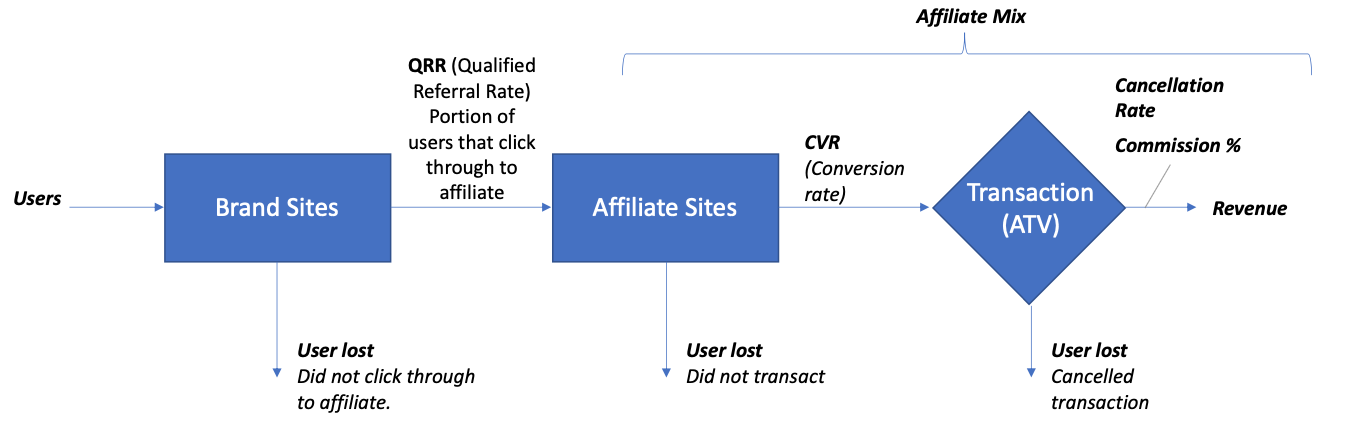
Simplified Business Model of an Affiliate Marketplace

Simplified Math Equation of an Affiliate Marketplace
4. Impossible goal
Consumer-facing businesses want to make a chatbot that reduces staffing costs. E-commerce shops would like to anticipate what their visitors are going to buy and target accordingly. Pharmaceutical companies would love to make a drug for cancer. After years of executing data science projects, we’ve noticed a trend. The chance of success appears to be related to the complexity of the problem. The more complex, the less likely it is to succeed.

The more complex, the higher chance of failure!
Solution: Simple is better
Partnering with a consultant or an agency that understands how to make the complex simple is a great way to increase success. And POWER SHIFTER is the expert here. As big believers (and practitioners) in simplifying digital experiences, they can attest to the importance of choosing simple projects. “What I’ve seen time and time again is that the right idea is always the simplest,” said JP Holeka, CEO & Founder of POWER SHIFTER. If your company’s BHAG (Big Hairy Audacious Goal) is to create a chatbot that reduces staffing costs, break it down into smaller steps.
Thriving in uncharted waters
AI and ML are being positioned as the next great step forward for humanity—but we have a ways to go before society realizes the full benefit of these powerful technologies. Because of the space's newness, companies looking to dive into AI and ML projects are navigating uncharted waters. If your organization doesn't have the in-house expertise, time, resources, or methodical R&D process, we encourage you to hire outside consultants early on to get going in the right direction. All projects come with a risk of failure, but you can amplify your chances of success with the right team.